The Ultimate Guide to Machine Learning Labeling Tools
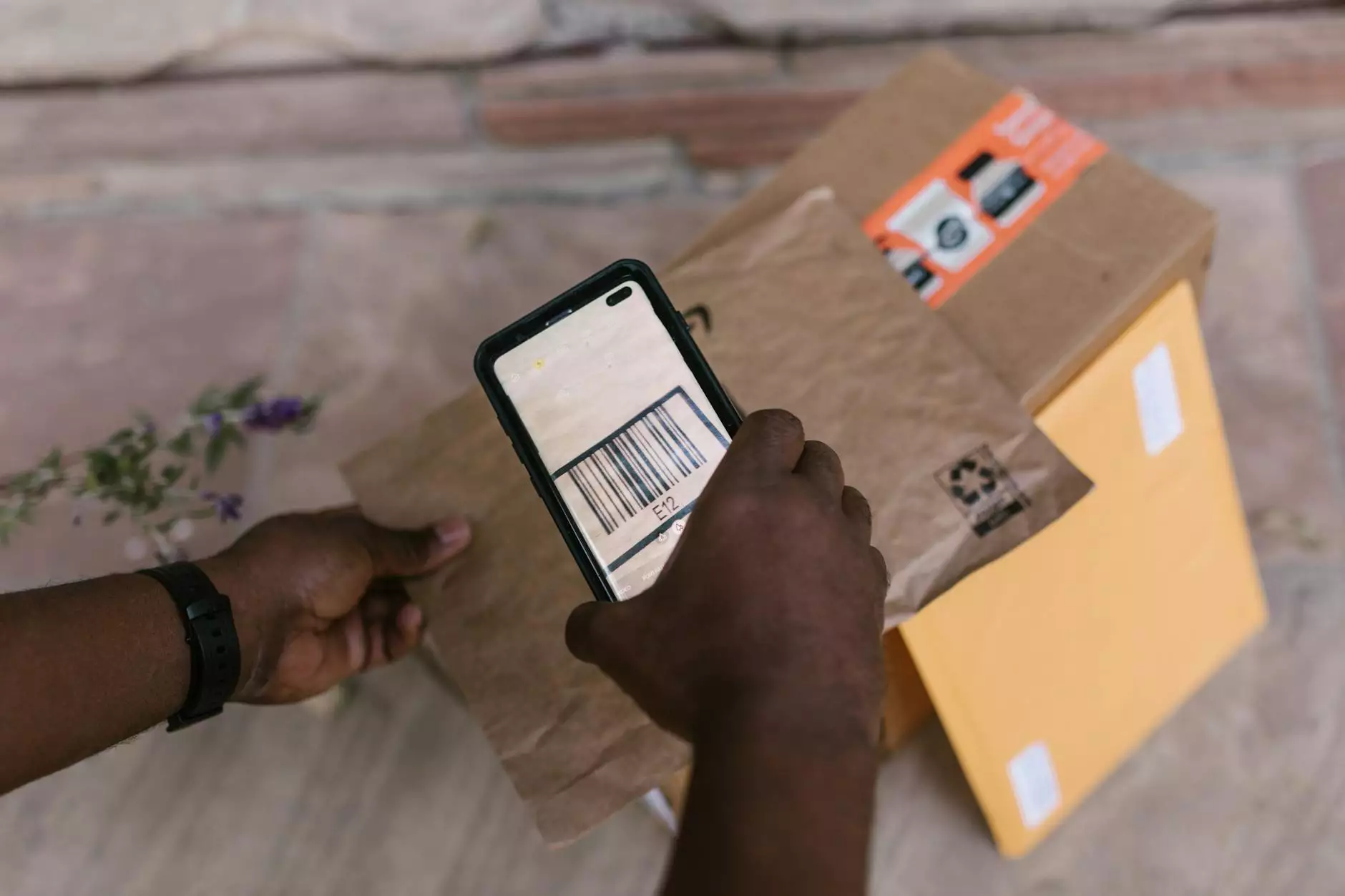
In the age of artificial intelligence and big data, the use of machine learning labeling tools has become paramount for businesses aiming to harness the power of data. Data is often chaotic and unstructured, requiring rigorous organization and labeling to be useful in training AI models. In this comprehensive guide, we’ll explore the various aspects of machine learning labeling tools, their significance in data annotation, and how platforms like Keylabs.ai are revolutionizing the approach to data preprocessing.
Understanding Machine Learning Labeling Tools
Machine learning labeling tools are software applications designed to classify, tag, and annotate data. These tools enable data scientists and machine learning engineers to prepare datasets for training algorithms. The data labeling process can involve several data types, including images, text, audio, and video. Each data type requires a different approach, making the choice of labeling tool crucial.
The Importance of Data Annotation
Data annotation is the foundational step in building effective machine learning models. Annotations provide context and meaning to raw data, making it interpretable for algorithms. Here’s why data annotation is essential:
- Improved Model Accuracy: A well-labeled dataset significantly enhances the quality and accuracy of the machine learning model.
- Behavioral Insights: Annotations allow data scientists to understand patterns and behaviors present in the data, which can lead to more insightful results.
- Error Reduction: Quality data annotation reduces the risk of errors in model predictions, ensuring more reliable outcomes.
Types of Data Annotation
Data annotation can be categorized based on the data types involved. Each category necessitates different labeling techniques:
1. Image Annotation
Image annotation is crucial for computer vision tasks such as object detection, image segmentation, and facial recognition. Techniques used in image annotation include:
- Bounding Boxes: Drawing rectangles around objects in images.
- Polygonal Segmentation: Outlining objects precisely with polygons.
- Landmark Annotation: Marking key points on an object.
2. Text Annotation
Text annotation involves tagging textual data to allow natural language processing (NLP) algorithms to understand it. Popular techniques include:
- Entity Recognition: Identifying named entities in a text, such as names, dates, and locations.
- Sentiment Analysis: Classifying the emotional tone behind a series of words.
3. Audio Annotation
For applications like voice recognition and speech analysis, audio annotation is vital. Techniques include:
- Transcription: Converting spoken words into written text.
- Speaker Identification: Labeling who is speaking in audio clips.
4. Video Annotation
Video annotation requires labeling moving objects over time, which is crucial for tasks involving object tracking and behavior analysis. Methods include:
- Activity Recognition: Identifying actions or behaviors within video clips.
- Frame-by-Frame Annotation: Annotating each frame of a video for precise identification of events.
Choosing the Right Machine Learning Labeling Tool
With an array of options available, selecting the right machine learning labeling tool is critical. Here are important factors to consider:
1. Data Type Compatibility
Ensure the tool supports the specific type of data you are working with. Some tools specialize in image labeling, while others might be tailored for text or audio.
2. User Interface and Usability
A user-friendly interface can drastically reduce the time spent on data labeling. Tools that are intuitive and easy to navigate enhance productivity.
3. Scalability
As your data grows, your labeling tool should be able to scale accordingly. Look for tools that can handle increasing volumes without compromising on performance.
4. Collaboration Features
In team scenarios, collaborative features such as real-time editing, commenting, and user roles are beneficial for improving workflow and communication.
5. Automation Features
Some machine learning labeling tools offer automated annotation capabilities, which can significantly speed up the process and reduce manual workload.
Keylabs.ai: A Leader in Data Annotation
When it comes to selecting a machine learning labeling tool, Keylabs.ai stands out as a leading provider of data annotation solutions. Here’s why:
Comprehensive Platform
Keylabs.ai offers a robust data annotation platform capable of handling various data types, including images, text, audio, and video. This versatility makes it an ideal choice for businesses with diverse projects.
Advanced Automation
The platform integrates cutting-edge automation features that streamline the labeling process. Automated tools help accelerate project timelines and reduce human errors, ensuring higher quality annotations.
User-Centric Design
With a focus on user experience, Keylabs.ai’s interface is designed to be intuitive. Users can easily navigate through tasks, making the annotation process efficient and straightforward.
Collaboration and Scalability
The collaborative features allow multiple users to work on projects simultaneously, facilitating seamless communication and teamwork. Furthermore, as businesses grow, Keylabs.ai scales effortlessly to meet increasing data demands.
Quality Assurance
Keylabs.ai emphasizes the importance of high-quality annotations. The platform incorporates quality assurance processes to validate the accuracy of labeled data, ensuring that the AI models trained on this data perform optimally.
Conclusion: Transforming Data with Machine Learning Labeling Tools
In conclusion, machine learning labeling tools are essential for any business looking to make the most of its data. With the proper data annotation platform, like Keylabs.ai, organizations can enhance their machine learning projects, leading to more accurate models and better decision-making.
By investing in high-quality data annotation, companies can transform chaotic data into valuable insights and capabilities. As the field of AI continues to evolve, mastering the art of data labeling will undoubtedly be a determining factor in the success of future AI initiatives. Embrace the potential of data with the best labeling tools and lead your business into a data-driven future.